
Unveiling the Impact of Artificial Intelligence in Forensic Science
Forensic science, the meticulous art of unraveling mysteries and solving crimes, stands as a formidable pillar in the pursuit of justice. In a world increasingly marked by technological advancements, the integration of Artificial Intelligence (AI) into forensic practices heralds a transformative era. This convergence promises to revolutionize the traditional methodologies, enhancing the precision, efficiency, and scope of forensic investigations. As we embark on a journey through the intricate tapestry of AI and forensic science, it becomes imperative to explore the profound implications, challenges, and ethical considerations that accompany this synergy. The marriage of cutting-edge AI technologies with the age-old pursuit of truth holds the potential to redefine how we unravel the complexities of criminal cases and, in doing so, shapes the very fabric of our legal landscape. This exploration seeks to shed light on the multifaceted impact of AI in forensic science, examining its role in crime scene analysis, biometrics, DNA analysis, cyber forensics, predictive policing, and the ethical dimensions that accompany this technological frontier. In essence, we delve into a realm where artificial intelligence becomes the silent yet potent ally of justice, transcending the boundaries of conventional investigative practices.
AI in Crime Scene Analysis
In the intricate dance of forensic science, crime scene analysis serves as the initial choreography, setting the stage for the investigation to unfold. Artificial Intelligence (AI) has gracefully waltzed into this critical phase, orchestrating a symphony of algorithms that redefine how we scrutinize and interpret crime scenes.
Automated Evidence Collection and Processing:
Traditionally, the painstaking task of evidence collection and processing has relied on the keen eyes and meticulous hands of forensic experts. Enter AI, equipped with computer vision algorithms capable of swiftly scanning crime scenes, identifying potential evidence, and even predicting patterns that might escape human observation. For instance, automated drones with AI-powered image recognition can navigate crime scenes, capturing data efficiently and minimizing the risk of oversight.
Improved Accuracy and Efficiency:
One of the primary virtues AI brings to crime scene analysis is its ability to process vast amounts of data swiftly. Machine learning algorithms can analyze patterns, recognize anomalies, and draw connections that might elude human cognition. Take gunshot acoustic analysis, for example. AI algorithms can discern subtle variations in sound patterns, helping investigators triangulate the origin of gunshots more accurately than traditional methods.
Example:
In a high-profile case, the integration of AI-driven analysis of surveillance footage played a pivotal role in identifying a suspect. The algorithm, trained to recognize distinctive gait patterns, pinpointed a masked individual who had managed to elude human recognition. This breakthrough underscored the potential of AI in transforming the efficiency of crime scene investigations.
As AI continues to refine its role in crime scene analysis, the synergy between technological precision and human intuition promises a future where the unraveling of criminal mysteries becomes a more streamlined and accurate endeavor. However, this integration is not without its challenges, as ethical considerations and potential biases in algorithmic decision-making demand a careful balancing act in the quest for justice.
Facial Recognition and Biometrics
The human face, with its intricate details, expressions, and unique features, has long been a focal point in forensic investigations. Artificial Intelligence (AI) has revolutionized this aspect through the lens of facial recognition and biometrics, reshaping how law enforcement identifies and tracks individuals.
Application of AI-driven Facial Recognition Technology:
AI-driven facial recognition technology has emerged as a formidable tool in the law enforcement arsenal. Facial recognition algorithms analyze facial features, creating distinct biometric profiles that can be matched against databases of known individuals. This technology extends beyond mere visual identification, incorporating deep learning techniques that enable machines to recognize faces under varying conditions, from different angles to changing lighting environments.
The precision of AI in facial recognition has significantly enhanced the identification process, expediting the search for suspects and aiding in the identification of victims. Machine learning models can match faces with a high degree of accuracy, even when dealing with large datasets. This capability proved invaluable in cases where traditional methods might have struggled to establish identity, contributing to a swifter resolution of criminal investigations.
*Example:*
A notable case involved the apprehension of a criminal suspect through a crowded city using AI-powered facial recognition on public surveillance cameras. The algorithm, trained to identify subtle facial features, successfully matched the suspect’s face against a criminal database, leading to a timely arrest. This instance underscores the potential benefits of AI in rapidly identifying individuals in dynamic and crowded environments.
Cyber Forensics and AI
In the era of digitization, where the battleground extends into the virtual realm, the significance of cyber forensics cannot be overstated. As crimes transcend physical boundaries and manifest in the intricate web of cyberspace, Artificial Intelligence (AI) emerges as a formidable ally in the quest to decipher the complexities of digital investigations.
Role of AI in Investigating Cybercrimes:
The proliferation of cybercrimes, ranging from hacking and identity theft to ransomware attacks, necessitates a sophisticated approach to digital forensics. AI, equipped with machine learning algorithms, proves indispensable in sifting through massive datasets, identifying patterns, and discerning anomalous activities indicative of malicious intent. For instance, anomaly detection algorithms can flag unusual user behavior or network traffic, providing early indications of a potential cyber threat.
Detection of Digital Evidence and Attribution:
Unraveling the digital trail left by cybercriminals often involves navigating through intricate layers of encryption and obfuscation. AI tools, including natural language processing algorithms and image recognition technologies, contribute to the extraction and analysis of digital evidence. Moreover, AI-driven attribution models assist in identifying the perpetrators behind cyberattacks by analyzing patterns, tactics, and techniques unique to specific threat actors.
Keeping Pace with Evolving Cyber Threats
The landscape of cyber threats is dynamic, characterized by constant evolution and sophistication. AI’s adaptive nature becomes a crucial asset in this context, enabling cybersecurity systems to learn from new threats and adjust their defense mechanisms accordingly. Threat intelligence platforms powered by AI can autonomously gather and analyze information, providing organizations with proactive insights into emerging threats.
Example:
In a recent cyber attack investigation, AI-based malware analysis tools played a pivotal role in dissecting a complex strain of ransomware. The algorithm not only identified the encryption patterns used by the malware but also traced its origin through a network of anonymized servers. This swift and precise analysis not only facilitated the recovery of encrypted data but also led to the apprehension of the cybercriminals involved.
Predictive Policing and AI
Predictive policing, a concept at the intersection of law enforcement and data analytics, has undergone a significant metamorphosis with the advent of Artificial Intelligence (AI). This section delves into the intricate landscape of predictive policing, exploring the advancements, controversies, and the delicate equilibrium between public safety and privacy concerns.
Overview of Predictive Policing Algorithms:
AI-driven predictive policing relies on complex algorithms that analyze historical crime data to identify patterns, trends, and potential hotspots. Machine learning models, often powered by vast datasets, aim to predict where and when crimes are likely to occur. These predictive models range from simple statistical tools to sophisticated artificial neural networks, capable of discerning intricate correlations within the data.
Controversies Surrounding the Use of AI in Law Enforcement:
The integration of AI in predictive policing has sparked debates surrounding privacy, civil liberties, and the potential perpetuation of biases inherent in historical crime data. Critics argue that relying on such data may exacerbate existing disparities and lead to discriminatory practices. Additionally, concerns about transparency and accountability in the decision-making processes of these algorithms have become focal points in the discourse on the ethical use of AI in law enforcement.
Balancing Public Safety and Privacy Concerns:
While the potential benefits of predictive policing include the allocation of resources more efficiently and the prevention of crimes, the delicate balance between public safety and privacy remains a critical challenge. Striking a harmonious equilibrium involves implementing safeguards such as regular audits, transparency in algorithmic decision-making, and ongoing efforts to mitigate biases in training datasets.
Challenges and Ethical Considerations
As we navigate the uncharted territory where Artificial Intelligence (AI) and forensic science intersect, we must confront a landscape fraught with challenges and ethical considerations. While the promises of technological advancement are alluring, the responsible integration of AI into forensic investigations demands a critical examination of the potential pitfalls and moral quandaries that accompany this evolution.
Addressing Biases in AI Algorithms:
One of the foremost challenges in deploying AI within forensic science lies in mitigating biases embedded within algorithms. Machine learning models are only as unbiased as the data on which they are trained. In the context of criminal justice, historical biases within datasets can inadvertently perpetuate existing disparities. For example, if an AI model is trained on arrest records that reflect societal biases, it may perpetuate or even exacerbate these biases, leading to unfair outcomes.
To address this, ongoing research focuses on developing techniques to identify and rectify biases within AI models. Explainable AI (XAI) methodologies aim to provide transparency, enabling forensic experts to understand how AI arrives at its conclusions and allowing for the identification and rectification of biased decision-making.
Potential Misuse of AI in Forensic Investigations:
The power vested in AI tools also raises concerns about potential misuse, either intentionally or unintentionally. As AI algorithms become integral to the decision-making process, there is a risk of over-reliance on these tools, leading to a diminished role for human expertise. Moreover, adversaries might exploit vulnerabilities in AI systems to manipulate evidence or compromise the integrity of forensic investigations.
To counter such threats, robust cybersecurity measures must be implemented to safeguard AI systems used in forensic science. Continuous monitoring and updating of AI algorithms to counter emerging threats become imperative, ensuring that the benefits of technological innovation are not overshadowed by security vulnerabilities.
Ensuring Ethical Standards in AI Tools:
The ethical dimension of deploying AI in forensic science cannot be overstated. The development, deployment, and use of AI tools must adhere to strict ethical standards to prevent unintended consequences. For instance, the use of facial recognition technology in law enforcement has sparked debates about privacy invasion and potential civil liberties infringements.
Ethical frameworks, guided by international standards and legal regulations, are essential to govern the ethical use of AI in forensic investigations. Public discourse and involvement in shaping these frameworks become crucial to ensure that societal values and concerns are taken into account.
Future Trends and Developments
As we navigate the complex landscape of Artificial Intelligence (AI) in forensic science, the horizon unfolds with promising innovations and potential breakthroughs. The symbiotic relationship between technological advancements and forensic investigations propels us toward a future where AI not only augments current practices but also paves the way for unprecedented methodologies.
Advancements in AI Technology for Forensic Science:
The relentless evolution of AI technologies continues to push the boundaries of what is achievable in forensic science. Quantum leaps in machine learning, natural language processing, and computer vision herald a future where AI algorithms become even more adept at deciphering intricate patterns within forensic data. Quantum computing, with its unparalleled processing capabilities, holds the promise of expediting complex analyses, enabling investigators to tackle large datasets with unprecedented speed.
Collaboration Between Researchers, Law Enforcement, and AI Developers:
The synergy between researchers, law enforcement agencies, and AI developers is becoming increasingly crucial in shaping the trajectory of AI in forensic science. Collaborative efforts bridge the gap between theoretical advancements and practical applications, ensuring that AI solutions are tailored to meet the specific needs and challenges faced by forensic investigators. Research institutions, police departments, and tech companies engage in partnerships to refine algorithms, conduct rigorous testing, and implement AI tools in real-world scenarios.
Potential Breakthroughs and Emerging Applications:
The landscape of potential breakthroughs is vast, encompassing a myriad of applications that could redefine forensic investigations. Quantum cryptography holds promise in securing sensitive forensic data, protecting it from unauthorized access or tampering. Augmented reality (AR) and virtual reality (VR) applications could revolutionize crime scene reconstructions, providing investigators with immersive environments to analyze evidence and recreate scenarios. The integration of AI with blockchain technology offers the potential to create tamper-proof, transparent chains of custody for forensic evidence, enhancing the reliability of information presented in court.
Conclusion:
In the symphony of justice, the integration of Artificial Intelligence (AI) with forensic science emerges as a transformative crescendo, promising a future where the pursuit of truth is harmonized with technological precision. The journey through the impact of AI on crime scene analysis, biometrics, DNA forensics, cyber investigations, predictive policing, and the challenges encountered along the way has illuminated the profound implications of this alliance.
The application of AI in crime scene analysis, exemplified by automated evidence collection and processing, underscores its potential to revolutionize the efficiency and accuracy of investigations. The utilization of facial recognition and biometric technologies, coupled with advancements in DNA analysis, opens new frontiers in identifying suspects and victims. In the realm of cyber forensics, AI becomes a sentinel against evolving digital threats, while predictive policing algorithms strive to enhance law enforcement strategies.
Yet, this alliance is not without its ethical considerations and challenges. The potential for biases in AI algorithms, concerns about privacy, and the ethical use of predictive technologies necessitate careful consideration. Striking a balance between innovation and ethical standards is crucial to ensuring that AI serves as an ally in justice rather than a source of controversy.
Looking ahead, the collaborative efforts between researchers, law enforcement, and AI developers promise to yield further advancements. Quantum computing, augmented reality, and blockchain technologies present exciting possibilities for enhancing forensic capabilities. The example of an AI algorithm detecting subtle facial microexpressions serves as a testament to the potential breakthroughs on the horizon.
As we stand at the crossroads of technology and justice, the future beckons with the prospect of a more efficient, accurate, and comprehensive approach to forensic investigations. The journey through the impact of AI in forensic science invites us to navigate with prudence, ensuring that the pursuit of truth is guided by the principles of fairness, transparency, and ethical responsibility. In this unfolding narrative, the symphony of justice resonates with the harmonious integration of human intuition and technological precision, offering a promising cadence for the future of forensic science.
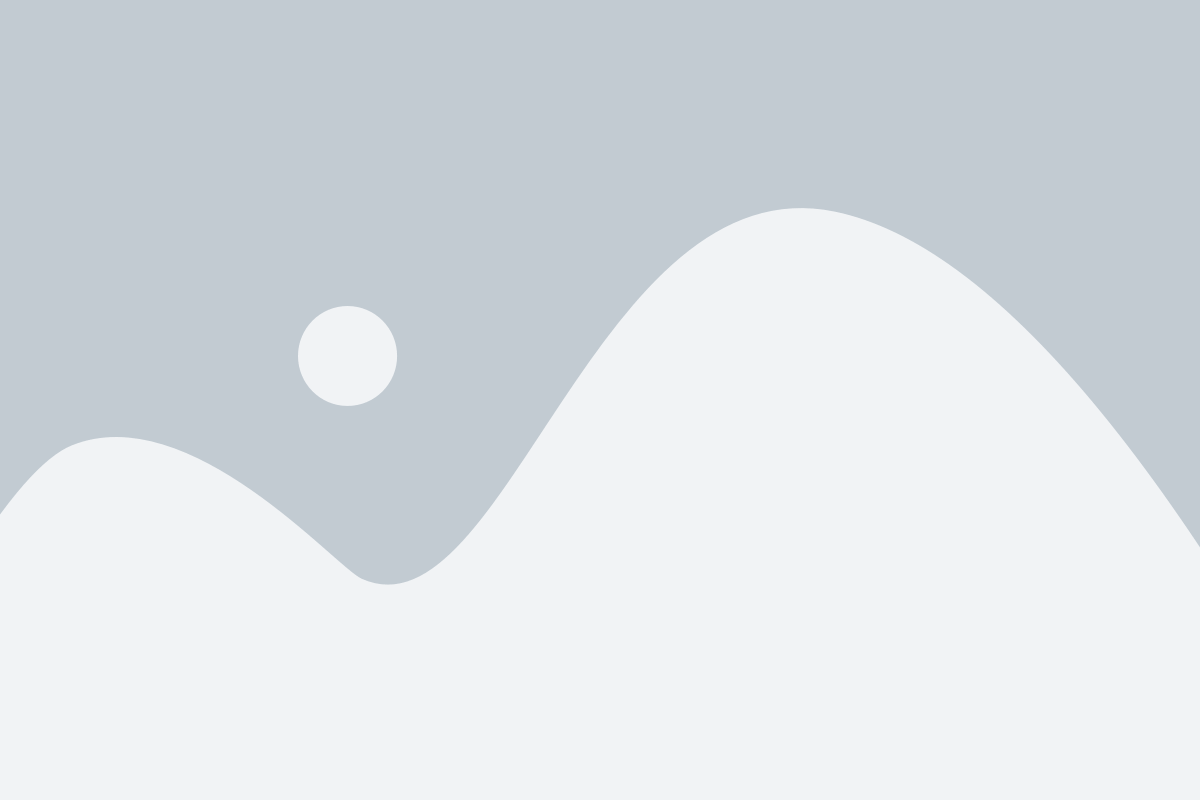
Mr. Shubham Saini-Assistant Professor
Forensic Science Department, Geeta University Panipat.
Related Posts
ETHICS OF TOURISM MANAGEMENT
Ethics play a crucial role in the management of tourism, influencing decision-making, sustainability, and the overall impact of tourism on destinations and communities. In this
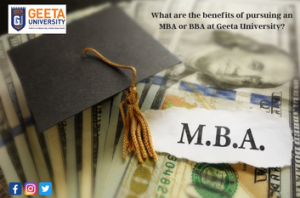
What are the benefits of pursuing an MBA or BBA at Geeta University?
What are the benefits of pursuing an MBA or BBA at Geeta University? An MBA or BBA degree from Geeta University can provide a number
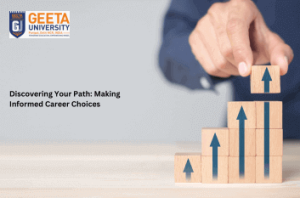
Discovering Your Path: Making Informed Career Choices – Geeta University
Discovering Your Path: Making Informed Career Choices – Geeta University Choosing a career path is an exciting yet challenging decision. With numerous options available, it’s