Understanding the Spread of Diseases through Mathematical Models
- August 20, 2024
- Geeta University
Mathematical modelling has emerged as a powerful tool in epidemiology, providing essential insights into the spread of diseases and informing public health decisions. These models use mathematical equations to represent the transmission dynamics of infectious diseases, enabling researchers and policymakers to predict outbreaks, evaluate control strategies, and understand the underlying mechanisms of disease spread.
This essay will explore the various types of mathematical models used in epidemiology, their applications in understanding disease transmission, and their critical role in guiding public health interventions.
Introduction to Mathematical Modelling in Epidemiology
Mathematical models in epidemiology are simplified representations of complex real-world processes. They help in understanding how diseases spread within populations and how various factors—such as transmission rates, recovery rates, and population structure—affect this spread. These models range from simple deterministic models to complex stochastic models that incorporate randomness and uncertainty.
The primary goal of these models is to provide a framework for analyzing the dynamics of infectious diseases, predicting future outbreaks, and assessing the potential impact of different intervention strategies. By simulating different scenarios, these models can help in making informed decisions to control and prevent the spread of diseases.
Types of Mathematical Models in Epidemiology**
Several types of mathematical models are commonly used in epidemiology, each with its strengths and limitations. The choice of model depends on the nature of the disease, the available data, and the specific questions being addressed.
a. Deterministic Models
Deterministic models assume that the outcome of the model is entirely determined by the initial conditions and parameters, without incorporating randomness. The most widely used deterministic model in epidemiology is the **SIR model**, which divides the population into three compartments:
Susceptible (S):Individuals who are not infected but are at risk of becoming infected.
Infectious (I): Individuals who are currently infected and can transmit the disease to susceptible individuals.
Recovered (R): Individuals who have recovered from the disease and are assumed to be immune.
The SIR model uses differential equations to describe the rate at which individuals move from one compartment to another. The basic SIR model can be extended to include additional compartments, such as exposed (E) individuals in the SEIR model, or vaccinated (V) individuals in the SIRV model
b. Stochastic Models
Stochastic models introduce randomness into the modeling process, allowing for the representation of uncertainty and variability in disease transmission. These models are particularly useful for small populations or in situations where the number of infections is low, and random events can significantly impact the outcome.
One common approach is the stochastic SIR model, which uses probabilities to determine the transitions between compartments. Stochastic models are often implemented using simulations, such as Monte Carlo simulations, to explore a wide range of possible outcomes.
c.Agent-Based Models
Agent-based models (ABMs) simulate the interactions of individual agents (e.g., people) within a population. Each agent follows specific rules regarding behavior, movement, and interactions with others. These models can capture complex, heterogeneous behaviors and provide detailed insights into how individual actions affect the overall dynamics of disease spread.
ABMs are particularly valuable for studying the impact of social networks, mobility patterns, and individual-level interventions (e.g., isolation, quarantine) on disease transmission. However, they are computationally intensive and require detailed data on individual behaviors and interactions.
d.Network Models
Network models represent the population as a network of nodes (individuals) connected by edges (contacts). These models are particularly useful for studying diseases that spread through close contact, such as sexually transmitted infections or respiratory diseases.
In network models, the structure of the network (e.g., how many contacts each individual has and how those contacts are distributed) plays a crucial role in determining the dynamics of disease spread. These models can help identify key individuals or groups (e.g., super-spreaders) who are critical for controlling the spread of the disease.
Applications of Mathematical Models in Understanding Disease Spread
Mathematical models have been instrumental in understanding various aspects of disease transmission and informing public health decisions. Here are some key applications:
Estimating the Basic Reproduction Number (R₀)
The basic reproduction number, R₀, is a critical parameter in epidemiology that represents the average number of secondary infections produced by one infected individual in a completely susceptible population. R₀ provides a threshold for determining whether an infectious disease will spread in a population.
If R₀ > 1, the disease is likely to spread, while R₀ < 1 indicates that the disease will eventually die out. Mathematical models are used to estimate R₀ based on the observed data and to explore how changes in transmission rates, recovery rates, or population structure might affect R₀.
Predicting the Course of an Outbreak
Mathematical models can predict the potential course of an outbreak, including the number of cases, the timing of the peak, and the total duration of the outbreak. These predictions are crucial for preparing healthcare systems, allocating resources, and implementing timely interventions.
For example, during the COVID-19 pandemic, mathematical models were used to predict the spread of the virus, the impact of public health measures (e.g., social distancing, lockdowns), and the potential consequences of different vaccination strategies.
Evaluating Intervention Strategies
Mathematical models allow researchers to evaluate the potential impact of various intervention strategies, such as vaccination, quarantine, social distancing, and treatment. By simulating different scenarios, models can identify the most effective strategies for controlling the spread of the disease.
For instance, models have been used to determine the optimal vaccination coverage needed to achieve herd immunity, the effectiveness of different quarantine policies, and the impact of contact tracing on reducing transmission.
Understanding Disease Dynamics in Heterogeneous Populations
Populations are often heterogeneous, with differences in age, immunity, behavior, and social networks. Mathematical models can incorporate these heterogeneities to provide a more accurate representation of disease dynamics.
For example, age-structured models can help understand how a disease might spread differently among children, adults, and the elderly, leading to more targeted interventions. Similarly, models that consider geographic heterogeneity can help identify regions at higher risk of outbreaks.
Modeling Emerging and Re-emerging Infectious Diseases
Mathematical models are crucial for understanding and responding to emerging and re-emerging infectious diseases, such as Ebola, Zika, and COVID-19. These models can provide early insights into the potential spread of the disease, identify critical parameters that need to be estimated, and guide the design of surveillance and control strategies.
During the early stages of an outbreak, models can help estimate key epidemiological parameters, such as the incubation period, transmission rate, and case fatality rate, which are essential for making informed public health decisions
Challenges and Limitations of Mathematical Models
While mathematical models are powerful tools, they are not without challenges and limitations. Some of the key challenges include:
Data Quality and Availability
The accuracy of mathematical models depends heavily on the quality and availability of data. In many cases, data on key parameters, such as transmission rates, contact patterns, and recovery rates, may be limited or unreliable, leading to uncertainty in model predictions.
Furthermore, data collection methods may vary across regions or over time, introducing additional biases that can affect the model’s accuracy. Addressing these challenges requires robust data collection and surveillance systems, as well as methods for dealing with uncertainty and incomplete data.
Model Assumptions and Simplification
Mathematical models often rely on assumptions and simplifications to make the modeling process tractable. However, these assumptions may not always accurately reflect the complexity of real-world disease dynamics.
For example, many models assume homogeneous mixing, where every individual has an equal chance of interacting with every other individual. In reality, contact patterns are often highly structured, with individuals interacting more frequently with certain groups (e.g., family, coworkers) than with others.
Similarly, models may assume constant parameters, such as transmission rates, over time, when in fact these parameters may change due to factors like seasonality, behavior changes, or the evolution of the pathogen
Interpretation and Communication of Results
Interpreting and communicating the results of mathematical models can be challenging, especially when dealing with complex models or uncertain predictions. It is essential for modelers to clearly communicate the assumptions, limitations, and uncertainties associated with their models to ensure that policymakers and the public understand the implications of the results.
Moreover, the results of mathematical models should be used as one of many tools in decision-making, rather than being viewed as definitive predictions. Models provide valuable insights, but they should be considered alongside other forms of evidence, such as empirical data, expert judgment, and ethical considerations.
The Role of Mathematical Models in Public Health Decision-Making
Despite their challenges, mathematical models play a crucial role in public health decision-making. They provide a systematic framework for analyzing the spread of infectious diseases and evaluating the potential impact of different interventions.
During public health emergencies, such as pandemics, mathematical models are often used to guide critical decisions, such as when to implement or lift lockdowns, how to allocate limited resources (e.g., vaccines, ventilators), and how to prioritize populations for interventions.
Models also play a key role in long-term planning, such as developing vaccination strategies, designing surveillance systems, and preparing for future outbreaks. By providing insights into the potential outcomes of different scenarios, mathematical models help policymakers make informed decisions that can save lives and reduce the burden of infectious diseases
Case Studies: Applications of Mathematical Models in Recent Outbreaks
To illustrate the impact of mathematical models in public health, here are some notable case studies:
The COVID-19 Pandemic
The COVID-19 pandemic highlighted the importance of mathematical models in guiding public health responses. Early in the pandemic, models were used to estimate the potential spread of the virus, predict healthcare demands, and evaluate the effectiveness of interventions such as social distancing, mask-wearing, and vaccination.
For example, the Imperial College COVID-19 Response Team developed a series of models that informed government decisions in the UK and other countries. These models provided estimates of the number of infections, hospitalizations, and deaths under different intervention scenarios, helping to shape national lockdown policies.
As the pandemic evolved, models continued to play a crucial role in assessing the impact of new variants, optimizing vaccine distribution, and planning for booster doses.
The 2014-2016 Ebola Outbreak in West Africa
During the 2014-2016 Ebola outbreak in West Africa, mathematical models were used to estimate the potential scale of the outbreak and guide international responses. Models helped identify the critical need for rapid interventions, such as isolation of cases, contact tracing, and safe burials, to prevent further spread.
One significant contribution came from the World Health Organization (WHO) and the U.S. Centers for Disease Control and Prevention (CDC), which used models to estimate the number of Ebola cases and deaths that could occur if effective interventions were not implemented. These estimates were instrumental in mobilizing resources and coordinating the international response.
Future Directions in Mathematical Modeling of Infectious Diseases
The field of mathematical modeling in epidemiology continues to evolve, driven by advances in data collection, computational methods, and interdisciplinary collaboration. Some emerging trends and future directions include:
Integration of Big Data and Real-Time Data Sources
The availability of large datasets, such as electronic health records, social media data, and mobile phone data, offers new opportunities for real-time modeling and surveillance. Integrating these data sources into mathematical models can improve the accuracy and timeliness of predictions, allowing for more responsive public health interventions.
For example, mobile phone data can be used to track population movements and estimate contact patterns, while social media data can provide insights into public behavior and sentiment during an outbreak.
Development of Multi-Scale and Multi-Disease Models
Many infectious diseases are influenced by factors operating at different scales, from individual-level behaviors to population-level dynamics. Developing multi-scale models that integrate these different levels of analysis can provide a more comprehensive understanding of disease transmission.
Similarly, multi-disease models that consider the interactions between different infectious diseases (e.g., co-infections, competition, or synergistic effects) can help in understanding complex epidemiological patterns and informing integrated public health strategies.
Conclusion
Mathematical models are indispensable tools in the fight against infectious diseases. They provide a framework for understanding the dynamics of disease spread, predicting outbreaks, and evaluating the effectiveness of public health interventions. By integrating data, theory, and simulation, these models offer valuable insights that guide decision-making and help protect public health.
However, the power of mathematical models also comes with responsibility. Modelers must carefully consider the assumptions, limitations, and uncertainties of their models and communicate these clearly to policymakers and the public. As the field continues to evolve, ongoing collaboration between modelers, epidemiologists, public health professionals, and policymakers will be essential for maximizing the impact of mathematical models in controlling infectious diseases and improving global health outcomes.
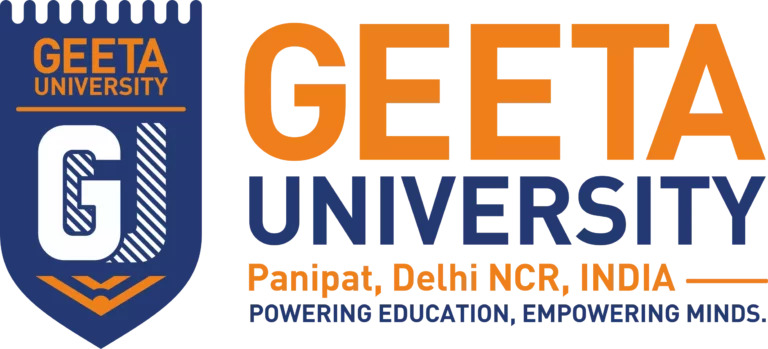
Admission Open 2024-2025
For Your bright Future
Tags
Related Posts
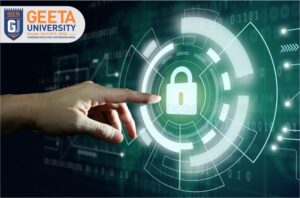
What is Data Security? | Why is Data Security important? | Types of Data Protection – Geeta University
For a variety of reasons, data security is critical for both public and commercial enterprises. First, there is the legal and ethical obligation of companies to protect their user and customer data from falling into the wrong hands. What is
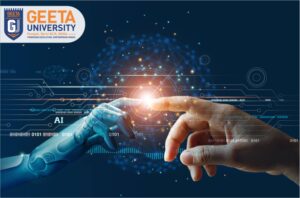
Artificial Intelligence: AI Terms Simply Explained – Geeta University
Unlike humans, AI learns and excels at a limited number of tasks. Weak or thin AI is the name given to such associate AI. They are currently typically superior to humans in their field. Artificial intelligence: AI Terms Simply Explained
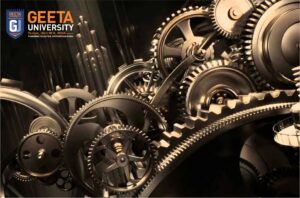
Career in Mechanical Engineering – Scope, Courses – Geeta University
Career in Mechanical Engineering- Are you dreaming of making a career in Mechanical Engineering? Do you want this information that how to become Mechanical Engineer. Career in Mechanical Engineering – Scope, Courses Scope of Mechanical Engineering Career in Mechanical Engineering-