Application of Correlation and Regression in Agriculture
- October 14, 2024
- Geeta University
Correlation and regression are powerful statistical tools used in various fields, including agriculture, to analyze relationships between variables, predict outcomes, and make informed decisions. In the agricultural sector, these techniques are essential for optimizing production, understanding environmental factors, improving crop yields, and enhancing resource management. This essay will explore the role of correlation and regression in agriculture, discussing their concepts, applications, and practical significance in agricultural research and practice.
1. Understanding Correlation and Regression
Correlation
Correlation is a statistical measure that quantifies the degree to which two variables are related. It is used to determine whether changes in one variable are associated with changes in another. The correlation coefficient (denoted as r) ranges from -1 to 1, where:
+1 indicates a perfect positive relationship (both variables move in the same direction).
-1 indicates a perfect negative relationship (one variable increases as the other decreases).
0 implies no linear relationship between the variables.
In agriculture, correlation is often used to examine relationships between different factors such as temperature, rainfall, soil fertility, crop yield, and other environmental or management variables.
Regression
Regression analysis goes a step further by not only measuring the strength and direction of the relationship between two variables but also modeling the relationship mathematically. It helps predict the value of one variable (the dependent variable) based on the known values of another variable (the independent variable).
There are various types of regression analysis, but the most commonly used in agriculture are linear regression (which models the relationship as a straight line) and multiple regression (which considers more than one independent variable).
2. Applications of Correlation in Agriculture
Understanding Climate and Crop Yield Relationships
Climate factors such as rainfall, temperature, and humidity are crucial determinants of crop yields. Correlation analysis helps determine how changes in these climatic variables are associated with variations in agricultural output. For instance, a strong positive correlation between rainfall and wheat yield in a specific region indicates that higher rainfall leads to better yields. This understanding can guide farmers in selecting crops based on expected weather conditions and managing irrigation needs effectively.
Soil Health and Crop Productivity
Soil fertility parameters, such as organic matter content, pH, and nutrient levels, have a direct impact on crop performance. Correlation analysis can help agronomists and soil scientists determine which soil properties are most strongly associated with crop yields. For example, high levels of nitrogen in the soil may have a positive correlation with maize yields. This information can be used to tailor fertilization strategies for optimal productivity.
Pest and Disease Incidence
The prevalence of pests and diseases in crops can often be correlated with environmental factors such as humidity and temperature. By studying the correlation between these factors, farmers and researchers can predict pest outbreaks and take preventive measures. For instance, a strong correlation between high humidity and the incidence of fungal diseases in crops can lead to the implementation of timely fungicide applications.
Water Management
Water is a critical resource in agriculture, and its availability can significantly impact crop production. Correlation analysis can be used to understand the relationship between irrigation levels and crop yield, helping farmers optimize water use. For example, a study might find a positive correlation between the amount of irrigation and rice yield up to a certain point, beyond which additional water may not significantly improve yield. This knowledge can prevent over-irrigation and conserve water resources.
3. Applications of Regression in Agriculture
Yield Prediction Models
Regression analysis is widely used in agriculture to develop yield prediction models. By analyzing historical data on factors such as temperature, rainfall, soil fertility, and farming practices, researchers can build models that predict future crop yields. These models are valuable for farmers and policymakers in planning for food production and managing resources. For instance, a linear regression model might predict wheat yields based on historical rainfall data, helping farmers make informed decisions about planting and harvesting.
Optimizing Fertilizer Use
Regression analysis helps in determining the optimal level of fertilizer application for different crops. By modeling the relationship between fertilizer use and crop yield, farmers can identify the point at which additional fertilizer no longer results in significant yield gains. This not only saves costs but also prevents environmental degradation from overuse of fertilizers. For example, a regression model could show that maize yield increases with nitrogen application up to a certain threshold, after which the marginal benefit diminishes.
Genotype-Environment Interaction
In plant breeding and genetics, regression analysis is used to study genotype-environment interactions. By analyzing data on the performance of different crop varieties across various environments, researchers can identify varieties that are best suited for specific conditions. This information is critical for developing new crop varieties that are resilient to environmental stresses such as drought or disease. For example, a regression model may be used to evaluate how different wheat varieties respond to varying levels of rainfall.
Economic Analysis of Farming Practices
Regression models are also employed in the economic analysis of farming practices. By analyzing data on input costs (such as seeds, fertilizers, labor) and output (crop yield or profit), researchers can determine which practices are most cost-effective. This helps farmers make better financial decisions. For instance, a multiple regression model could be used to evaluate the impact of seed variety, irrigation level, and fertilizer application on farm profitability.
4. Role of Multiple Regression in Complex Agricultural Systems
Agriculture is influenced by a wide range of factors that often interact in complex ways. Multiple regression analysis allows researchers to account for multiple variables simultaneously, providing a more comprehensive understanding of the relationships between inputs and outcomes.
Crop Growth Modeling
In crop growth models, multiple regression analysis is used to assess the combined effects of various factors such as temperature, soil moisture, and nutrient availability on plant growth. For instance, a multiple regression model may be developed to predict wheat growth based on variables such as temperature, soil pH, and nitrogen content. This helps in making precise recommendations for crop management.
Assessing Risk in Agriculture
Farmers face numerous risks, including price volatility, climate change, and pest outbreaks. Multiple regression can be used to model these risks by incorporating various factors that influence agricultural productivity. For example, a model might include variables such as market prices, input costs, and climate data to assess the economic risks faced by farmers in a particular region. This helps in developing risk management strategies, such as crop insurance programs or diversification of crops.
5. Challenges and Limitations
While correlation and regression are invaluable tools in agriculture, they come with limitations.
While correlation and regression are invaluable tools in agriculture, they do come with several limitations that need to be considered to avoid misinterpretation of results or misguided decisions. Below are some of the key limitations:
Correlation Does Not Imply Causation
One of the most significant limitations of correlation analysis is that it cannot establish causality between variables. Even if a strong correlation exists between two variables, this does not necessarily mean that one variable causes the other to change. For example, there may be a strong correlation between the use of fertilizers and crop yield, but this does not mean fertilizers alone are responsible for the yield increase—other factors like irrigation, weather, and pest control might also contribute.
In agricultural research, failing to recognize this distinction can lead to erroneous conclusions. It is essential to complement correlation analysis with experimental studies or further analysis to determine if there is a causal relationship between the variables.
Overfitting in Regression Models
Regression models, especially complex ones with many variables, are susceptible to **overfitting**. Overfitting occurs when the model captures not only the underlying relationship between variables but also the random noise present in the data. While this may result in a model that fits the historical data very well, it might perform poorly when used to predict new or unseen data.
In agriculture, this can be problematic when building models that are intended to generalize to other regions, crops, or time periods. For example, a regression model that accurately predicts maize yields based on data from one growing season in a specific location may not work well for a different season or region due to changes in environmental conditions or crop varieties.
Assumption of Linearity
Simple linear regression assumes that the relationship between the independent and dependent variables is linear, which means it fits a straight line to the data. However, many agricultural processes involve non-linear relationships. For instance, crop yields may initially increase with higher levels of irrigation but may plateau or even decline if excessive water leads to issues like waterlogging or nutrient leaching.
In such cases, the assumption of linearity can lead to misleading results, and more advanced techniques such as non-linear regression or polynomial regression may be required to accurately model the relationship.
Influence of Outliers
Outliers, which are data points that differ significantly from other observations, can disproportionately influence both correlation and regression results. In agricultural data, outliers may arise due to measurement errors, extreme weather events, or unusual farming practices. If not properly accounted for, these outliers can distort the analysis.
For example, an unusually high crop yield in one year due to exceptionally favorable weather might skew the results of a regression model, leading to overly optimistic predictions for future yields. In correlation analysis, outliers can lead to artificially high or low correlation coefficients, masking the true relationship between variables.
Multicollinearity in Regression Models
In multiple regression analysis, multicollinearity occurs when two or more independent variables are highly correlated with each other. This can cause problems in estimating the coefficients of the regression model, leading to unreliable or unstable estimates. In agriculture, many variables are naturally interrelated. For example, soil fertility, irrigation, and fertilizer application may all be highly correlated, making it difficult to determine the unique effect of each variable on crop yield.
When multicollinearity is present, it can be challenging to interpret the results of the regression analysis accurately. One way to address this issue is by using techniques such as principal component analysis (PCA) or ridge regression, which reduce the impact of multicollinearity.
Non-Stationary Data
Agricultural data often exhibit non-stationary characteristics, meaning that the statistical properties of the data change over time. For example, crop yields may improve over time due to technological advancements, changes in farming practices, or improved seed varieties. If the data are non-stationary, applying standard correlation and regression techniques may lead to misleading results, as these methods assume that the relationships between variables remain constant over time.
Time-series techniques, such as differencing or autoregressive integrated moving average (ARIMA) models, may be necessary to account for the evolving nature of agricultural data.
Extrapolation Beyond the Data Range
Regression models are often used to make predictions. However, extrapolating beyond the range of the data used to build the model can lead to unreliable predictions. For instance, a regression model that predicts wheat yield based on historical temperature data may not be reliable if used to predict yields under temperature extremes that were not present in the original dataset.
In agriculture, conditions can vary widely across seasons, regions, and climatic zones, making it risky to apply a model beyond the range of observed data. Researchers and practitioners should be cautious when using regression models to make predictions under conditions that are significantly different from those in the training data.
Omitted Variable Bias
Omitted variable bias occurs when a key variable that influences the dependent variable is left out of the regression model. In agriculture, there are often many factors influencing crop yields, including weather, soil conditions, pests, and farming practices. If an important variable is omitted, the regression model may produce biased results, leading to incorrect predictions or conclusions about the effects of the included variables.
For example, a regression model predicting crop yield based solely on fertilizer use, without accounting for irrigation levels, may overestimate the impact of fertilizers on yields. Proper model specification and inclusion of all relevant variables are crucial to avoid this bias.
Conclusion
Correlation and regression are fundamental tools in agricultural research and practice, helping to analyze relationships between variables, predict outcomes, and optimize resource use. They are widely applied in understanding climate effects, improving crop management, developing predictive models, and assessing economic risks. Despite their limitations, when used correctly, these statistical methods provide valuable insights that drive innovation and efficiency in agriculture.
In the face of challenges like climate change, food security, and environmental sustainability, the role of correlation and regression in agriculture will continue to grow, enabling farmers, scientists, and policymakers to make data-driven decisions that enhance productivity and resilience in agricultural systems.
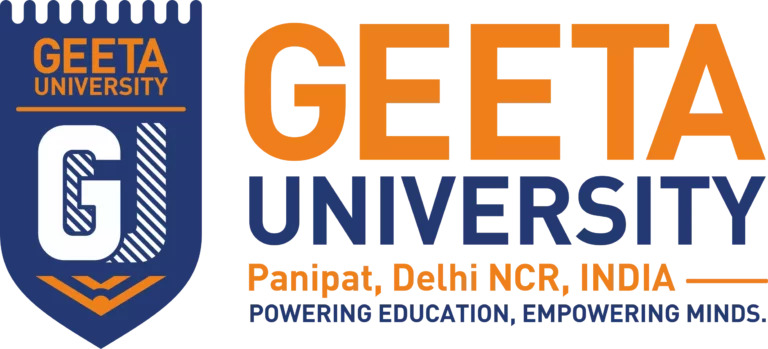
Admission Open 2024-2025
For Your bright Future
Heading
If you are a good listener and can provide solutions for people’s problems, a life coach is the perfect business idea for you. You can do this face to face, through a podcast, on YouTube or other video streaming platforms, etc.
We have shortlisted trendy business ideas for fresh MBA graduates. These ideas suit the respective best MBA courses. Most of these ideas do not require huge investment and ensure quicker ROI on the business. Start today your journey of entrepreneurship with one of these business ideas.
Heading
If you are a good listener and can provide solutions for people’s problems, a life coach is the perfect business idea for you. You can do this face to face, through a podcast, on YouTube or other video streaming platforms, etc.
We have shortlisted trendy business ideas for fresh MBA graduates. These ideas suit the respective best MBA courses. Most of these ideas do not require huge investment and ensure quicker ROI on the business. Start today your journey of entrepreneurship with one of these business ideas.
Heading
If you are a good listener and can provide solutions for people’s problems, a life coach is the perfect business idea for you. You can do this face to face, through a podcast, on YouTube or other video streaming platforms, etc.
We have shortlisted trendy business ideas for fresh MBA graduates. These ideas suit the respective best MBA courses. Most of these ideas do not require huge investment and ensure quicker ROI on the business. Start today your journey of entrepreneurship with one of these business ideas.
Heading
If you are a good listener and can provide solutions for people’s problems, a life coach is the perfect business idea for you. You can do this face to face, through a podcast, on YouTube or other video streaming platforms, etc.
We have shortlisted trendy business ideas for fresh MBA graduates. These ideas suit the respective best MBA courses. Most of these ideas do not require huge investment and ensure quicker ROI on the business. Start today your journey of entrepreneurship with one of these business ideas.
Heading
If you are a good listener and can provide solutions for people’s problems, a life coach is the perfect business idea for you. You can do this face to face, through a podcast, on YouTube or other video streaming platforms, etc.
We have shortlisted trendy business ideas for fresh MBA graduates. These ideas suit the respective best MBA courses. Most of these ideas do not require huge investment and ensure quicker ROI on the business. Start today your journey of entrepreneurship with one of these business ideas.
Conclusion
If you are a good listener and can provide solutions for people’s problems, a life coach is the perfect business idea for you. You can do this face to face, through a podcast, on YouTube or other video streaming platforms, etc.
We have shortlisted trendy business ideas for fresh MBA graduates. These ideas suit the respective best MBA courses. Most of these ideas do not require huge investment and ensure quicker ROI on the business. Start today your journey of entrepreneurship with one of these business ideas.
Tags
Related Posts
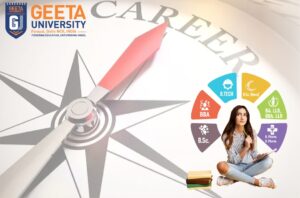
How to find the correct stream after the 10th standard? – Geeta University
Are you confused about which stream to choose after 10th? What is the best stream of the future? What should you learn after Class 10th? Almost all students sails in the same boat. These are the top questions asked by
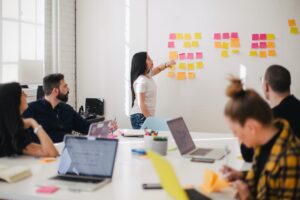
Best Courses After 12th Science, Future, Scope and Career Prospects – Geeta University
One question that keeps popping up in the minds of all 12th grade students is how to choose a safe, secure and fruitful career option. The task of choosing the right path can be difficult for Grade 12th students specially
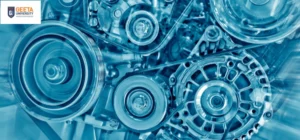
Best Courses After 12th Arts, Future, Scope and Career Prospects – Geeta University
It is important to choose the right subject for your graduation because it is probably the most important time for your study. Making the right decision today will put you on the path to school and professional success by enrolling